If you’ve ever asked yourself, “Does algorithmic trading work? “, you want real answers. Algorithmic trading uses complex algorithms to make trades automatically, which could lead to faster and more profitable results.
This article talks about how well algorithmic trading works in the real world, breaking down how to measure its success, how it depends on good strategies and how the market works, and the risks that come with it. We look at the facts, trends, and testimonies that show how and when algorithmic trading works, ignoring the claims that it always does.
Key Takeaways
- Algorithmic trading uses complicated mathematical models and high-frequency trading to run a methodical, data-driven process that is better than what humans can do.
- Algorithmic trading improves execution accuracy, speeds up trade frequency, and helps with risk management. However, it also comes with challenges and risks, such as the chance of technical errors and being responsible for losses when market conditions change quickly.
- In many financial markets, algorithmic trading is becoming more common and makes up a big part of trading volume. Hedge funds and institutional investors take advantage of its efficiency, but retail traders may find it hard to use because it’s hard to understand and costs a lot.
Understanding Algorithmic Trading
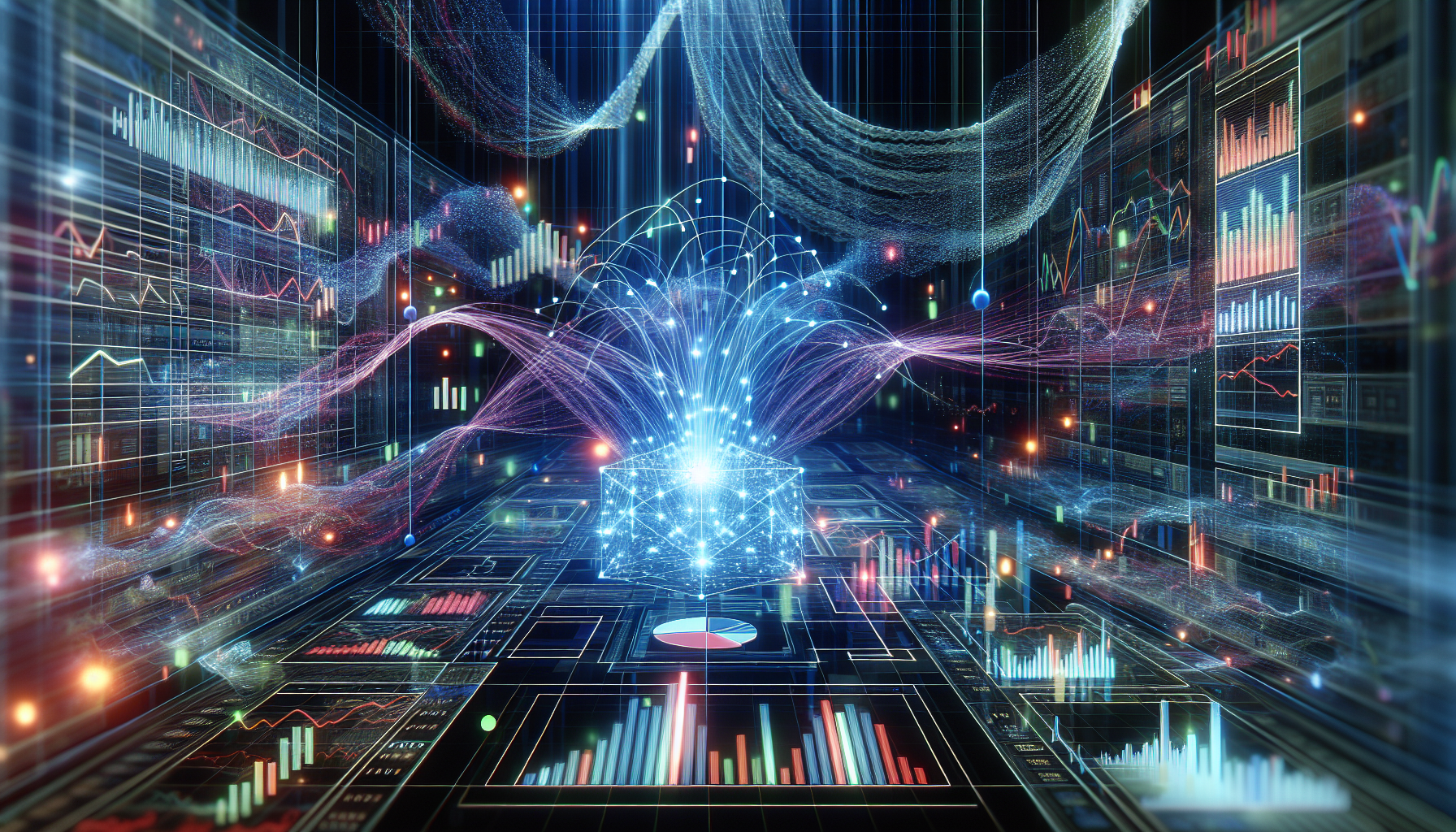
The way financial markets work has changed a lot because of algorithmic trading, in which computer programs make trading decisions. Using complex mathematical models, institutions and individuals, including algorithmic traders, can make tens of thousands of trades per second using the power of algo trading. This automated tapestry of trading strategies includes everything from high-frequency algorithms to execution strategies. Each is made to take advantage of a different part of the market.
In addition to being fast, algorithmic trading brings a systematic, data-integrated approach to the trading floor. It automates tasks and decisions that used to be the sole domain of humans.
The Role of Algorithms in Trade Execution
Putting trades into action with computer algorithms is like driving a Formula 1 car in a go-kart race—it changes everything. These algorithms automatically make trades based on set rules that take into account market conditions, price changes, and financial data to get the best results. They are programmed to make trades faster and more efficiently than humans can. To do this, they often use arrival price and implementation shortfall algorithms to keep market impact and execution costs as low as possible.
One thing that makes algorithm trading stand out is its unmatched speed and frequency, which are much higher than what humans can do.
Automated Trading System Dynamics
Modern technology is needed for an automated trading system to work. To manage and keep track of trading instruments, market data protocols, feed handlers, and data storage must all work together smoothly. Before being released into the wild markets, these systems are put through a lot of backtesting using both historical and real-time data to make sure they will make money.
Execution logic and risk management keep an eye on things to make sure orders are handled correctly and losses are kept to a minimum with stop-loss orders and position limits. Protocols for entering and following exchange orders are kept up to date by this high-tech orchestra. This cuts down on human error and lets the orchestra quickly respond to changes in the market.
Measuring the Success of Algorithmic Trading
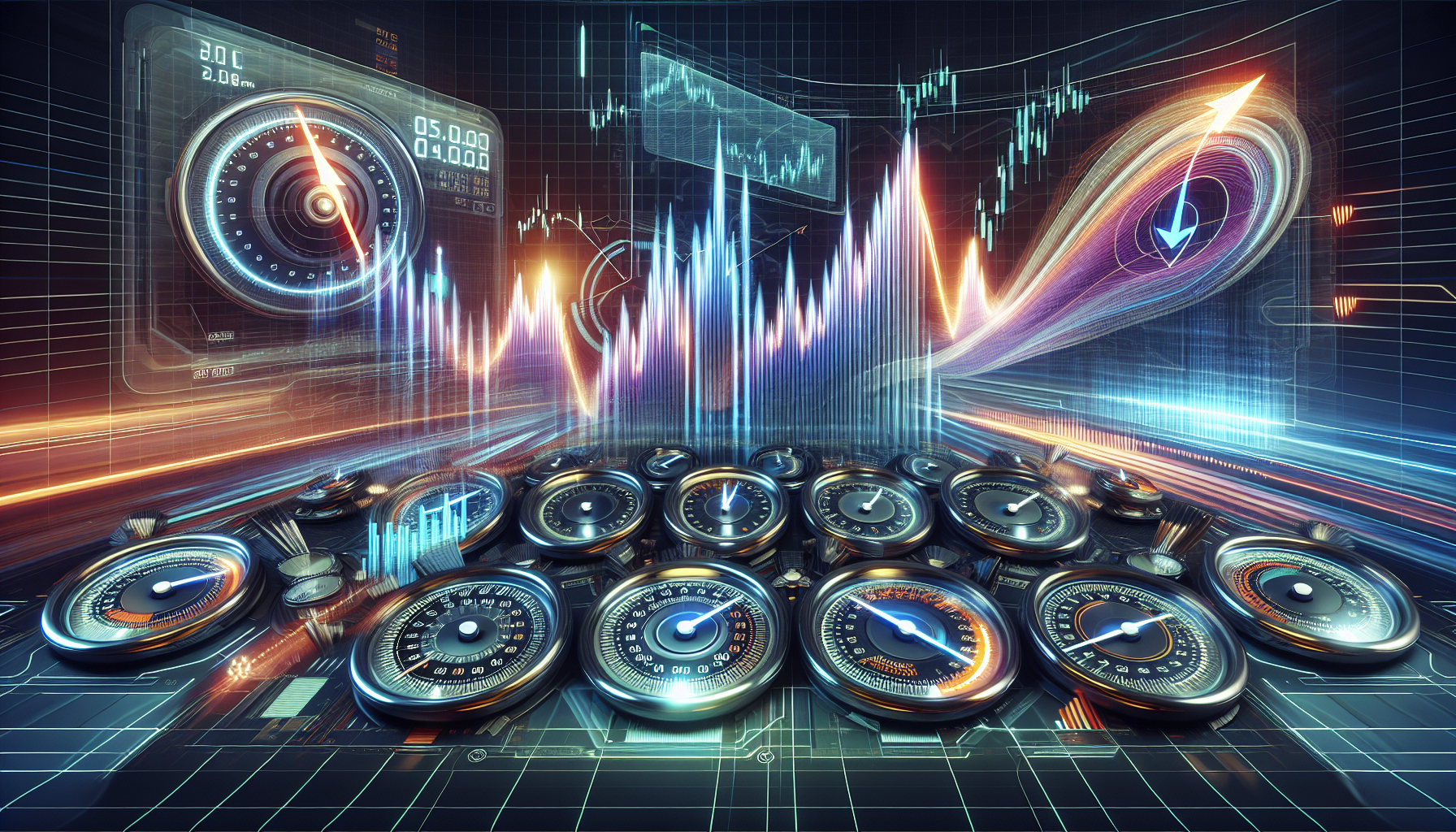
In algorithmic trading, success isn’t just about making money; it’s also about keeping up a good level of performance over time. The real value of an algorithm is shown by how fast and often it trades, how well it can get the best execution prices, and how well it can cut through transaction costs. For algorithmic traders, these are the standards that tell the difference between the average and the extraordinary.
Profitability Analysis
A lot of people, especially hedge funds and institutional investors, have made a lot of money with algorithmic trading. But for small investors, the climb is usually more difficult. Algo-trading gets rid of the emotional and transactional fat that slows down traditional trading by making trades at the best prices and times. Which strategy is used—trend-following, arbitrage, or mean reversion?—is very important to how profitable algorithmic trading is. Each strategy has its own pros and cons.
Some key points about algorithmic trading include:
- Hedge funds and institutional investors have been making a lot of money, which makes algorithmic trading profitable for them.
- When you trade, it cuts down on the emotional and transactional fat.
- The strategy you choose is very important for making money, and each strategy has its own pros and cons.
Algo-trading has been used by institutional investors to automatically carry out large orders. This has cut down on trade costs and increased profits. However, algorithmic trading comes with its own risks, just like any other type of trading. Strategies that are too focused on past data can fail when they try to use them in the real market, which is very unpredictable.
Market Impact and Volatility
A lot of the market’s activity is done by algorithmic trading strategies like VWAP and TWAP. They keep the market from being too affected by breaking up large orders and putting them in slowly. These strategies make order execution better, and because they are more subtle and have less of an effect on the market, they often do better than manual trading. The systematic nature of algorithmic trading makes the market more liquid, which makes it easier for prices to be found. But big algorithmic trades can still make things more volatile and unstable, even though they make the market more liquid.
When the market is volatile, strategies like rebalancing index funds can help you make money and lower your risk by relying on small, predictable trades. But volatility is still a strong enemy because algorithms can fail in markets that change quickly, which could cause big losses. Even though algorithmic trading can help with liquidity, it also has a weakness: mistakes in automated strategies can cause huge financial waves.
The Attraction of Algorithmic Trading Strategies
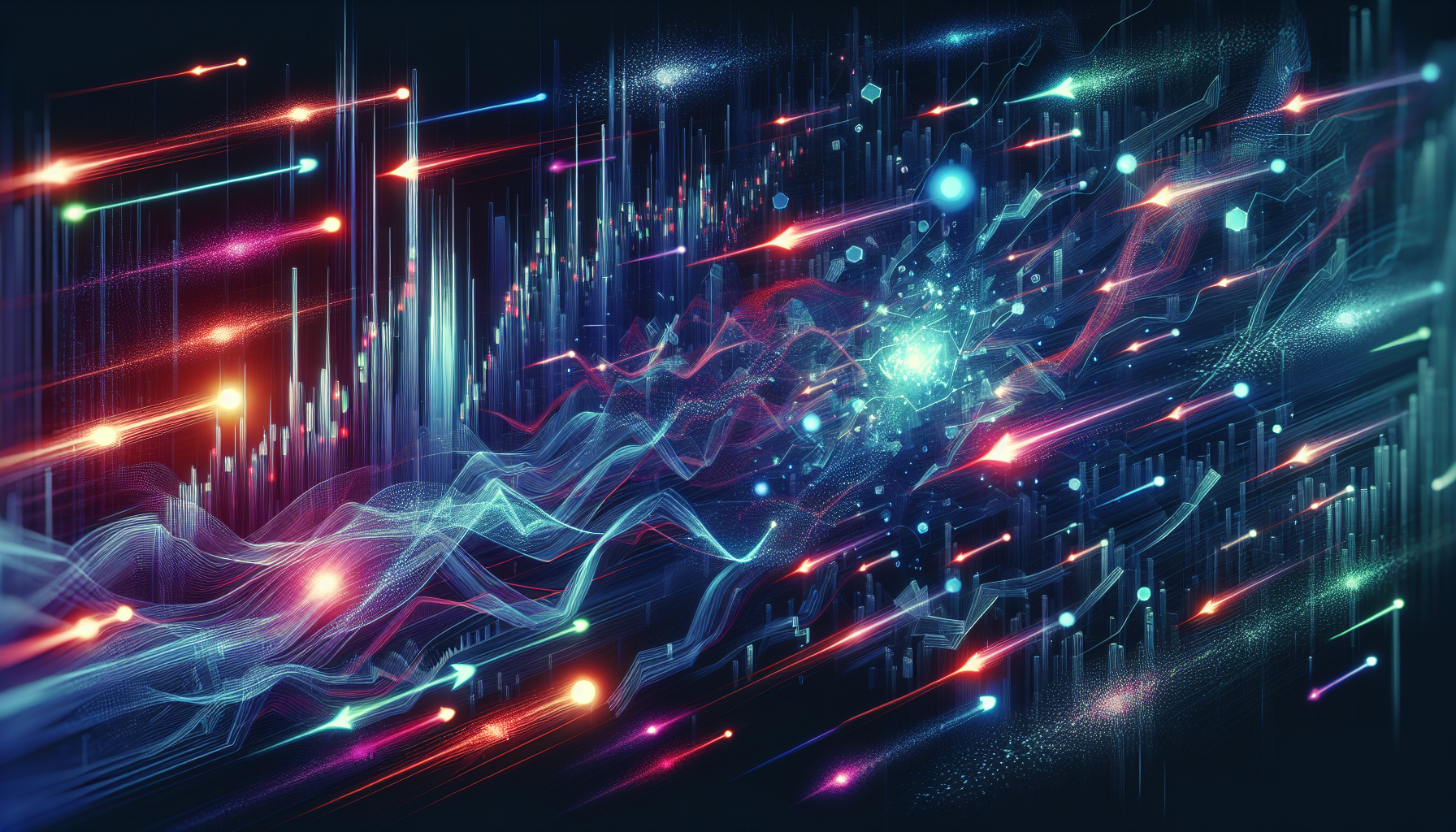
The allure of algorithmic trading strategies, with their promise of:
- precision
- speed
- risk management
- diversification
is undeniably enticing for traders. These strategies have laid claim to a significant portion of trading volume across the globe, ensuring systematic trading by dampening the emotionally charged whims of human decision-making. When algorithms are in charge, the chance of making mistakes by hand is much lower. This makes trading more disciplined and less emotionally charged.
The range of algorithmic trading strategies is very broad and deep. It includes everything from following trends to using mathematical models. The fact that these strategies have grown from simple rules in the 1970s to complex algorithms today shows how well they work and how technological progress has helped them do so.
Precision and Speed
High-frequency trading, or HFT, is the fastest and most accurate way to trade using algorithms. HFT has changed from milliseconds to microseconds, showing that it is always trying to do things perfectly. Algorithmic trading makes trades possible at speeds that beat human traders to the punch, letting them quickly take advantage of short-lived market opportunities.
The benefits of algorithmic trading include:
- The ability to make trades in multiple markets at the same time.
- Following strict trading rules and not letting your emotions affect your decisions.
- Improving the accuracy of trading.
Risk Management and Diversification
Serving as a beacon for risk management and diversification, algorithmic trading illuminates the path towards portfolio resilience. Algorithm trading spreads investments across many different types of assets, which might lower unsystematic risk. Being able to trade across multiple strategies and markets at the same time is like having a financial Swiss Army knife—it gives you a lot of tools to make money and lower your risk.
In algo-trading, automated algorithms are great at doing more than one thing at once. They can set up automated trading strategies that:
- You can look at and trade many things at once, like stocks and derivatives. This makes it easy to have a diversified investment strategy.
- Rebalance portfolios in real time to keep them at the level of diversification you want.
- Handle big orders in smaller pieces to get a feel for how they affect the market.
- Keep an eye on how markets and assets are linked and change investment spreads to get the best balance of risk and reward.
Because of these features, algorithmic systems are very useful and efficient in the trading world.
Besides executing trades, these systems offer the following advantages:
- The agility to modify or cancel orders nearly instantaneously, an invaluable asset in the dynamic landscape of risk management
- There are advanced algorithms that can help make trading less likely to go wrong.
- Strong risk management procedures that are needed to trade successfully
- Machine learning capabilities that analyze market data to calculate optimum asset allocations and position sizes, maximizing risk-adjusted returns
Machine learning is also a key part of optimizing portfolios, which means finding the best mix of assets to get the best returns while minimizing risk.
Algorithmic Trading Across Financial Markets
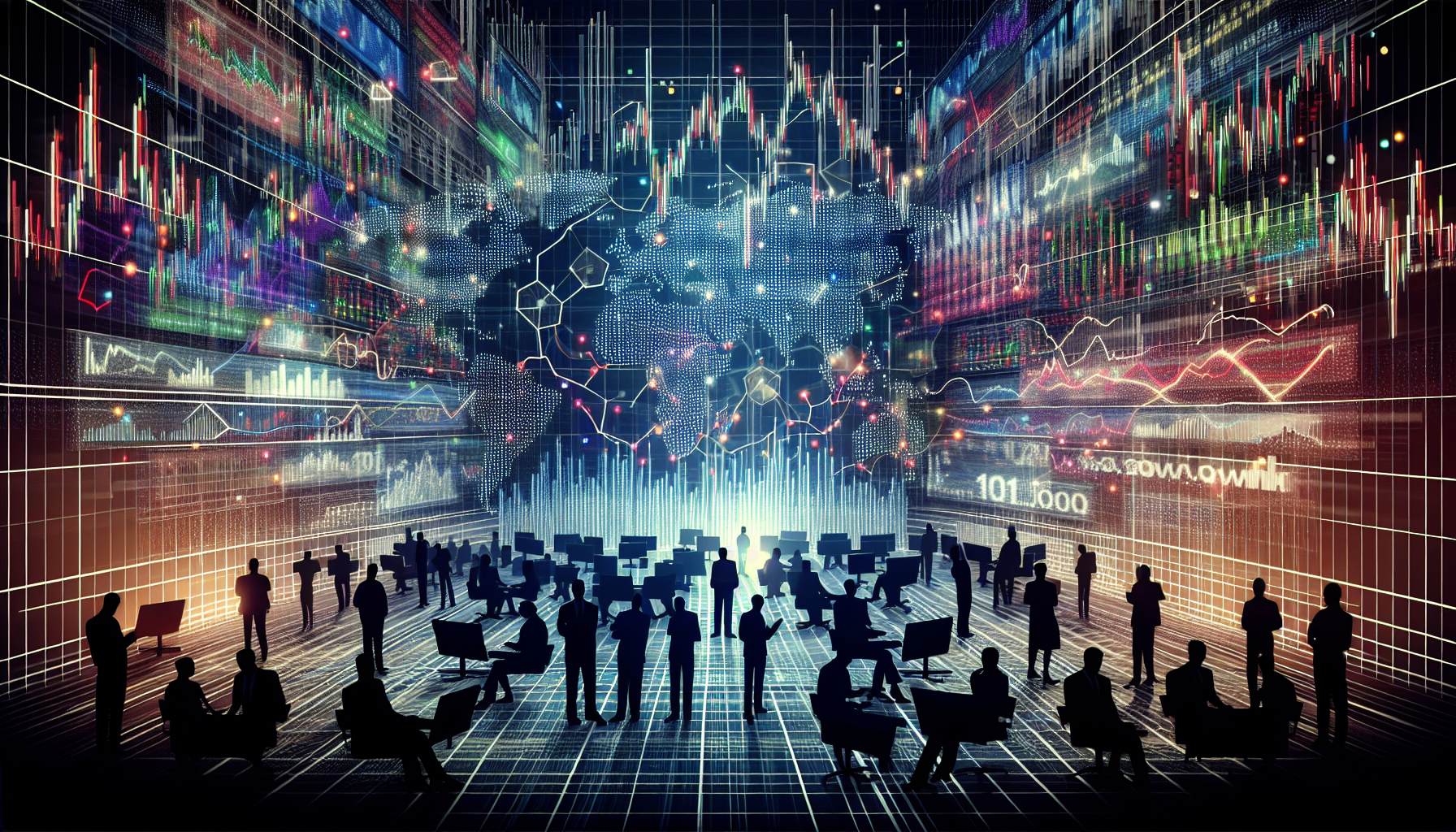
The financial markets are all affected by algorithmic trading. It makes up a big part of trading volume everywhere, from the busy U.S. stock market to markets in emerging economies like India. This growth around the world is due to new technology that has made automated trading more accessible to everyone, appealing to both large trading firms and individual traders.
As more and more exchanges around the world adopt these trading strategies, it becomes clear that they work well in today’s trading environment.
Equity and Futures Market Penetration
The stock and futures markets have been very open to algorithmic trading, which has seen a lot of growth over the years. Between 2021 and 2026, algorithmic trading in stocks is expected to grow at a rate of 11.23% per year. By 2027, this growth is expected to have added $8.61 billion to the market share of algorithmic trading.
These markets have become great places for automated trading, which makes up about 60% to 70% of all stock trading and 40% to 50% of all futures trading.
Foreign Exchange and Fixed-Income Markets
You can use algorithmic trading in the foreign exchange and fixed-income markets, and its importance is growing very quickly there. Fixed-income markets are becoming more electronic. This is happening because investments in technology, such as artificial intelligence, are pushing the limits of what is possible. For example, 34.4% of investment-grade bonds are traded electronically.
However, the higher rates of electronic adoption are still slowed down by the fact that markets are fragmented in places like the Asia-Pacific region and there are problems with standardization in Europe.
The Challenges of Algorithmic Trading for Retail Traders
When retail traders try to get into algorithmic trading, they often run into a maze of problems. Not having enough money can be a big problem because it keeps them from covering losses or paying for the infrastructure they need to build and run algorithms.
Individual traders may not be able to afford to set up an algorithmic trading system because of the high costs involved, such as ongoing fees for software and data feeds. Not knowing how to write trades and not having a mentor make these problems even worse because they are needed to improve strategies and find your way around the complicated trading world.
Developing Profitable Systems
To get from discretionary trading to automated trading, you have to make a big change that includes new technological and strategic areas. For algorithmic trading strategies to work, you need to know a lot about market microstructure because it is one of the most important factors that determines trading success.
When retail traders start using algorithms to trade, they have to deal with the complicated task of managing data, which includes putting together and formatting data from many different sources, each with its own unique identifiers.
Technical Glitches and System Failures
It’s possible for even the most advanced algorithmic trading systems to have technical problems and stop working. When these problems happen, they can have big effects on finances and make trading systems less effective. These are some common technical problems that can happen with algorithmic trading:
- Bugs in software
- Having trouble connecting
- Failures of hardware
- Not right trade executions
The flash crash of 2010 is a stark reminder of how technical problems in automated trading systems can make market problems worse. This shows how important it is to design and oversee systems well.
Advancements in Trading Algorithms
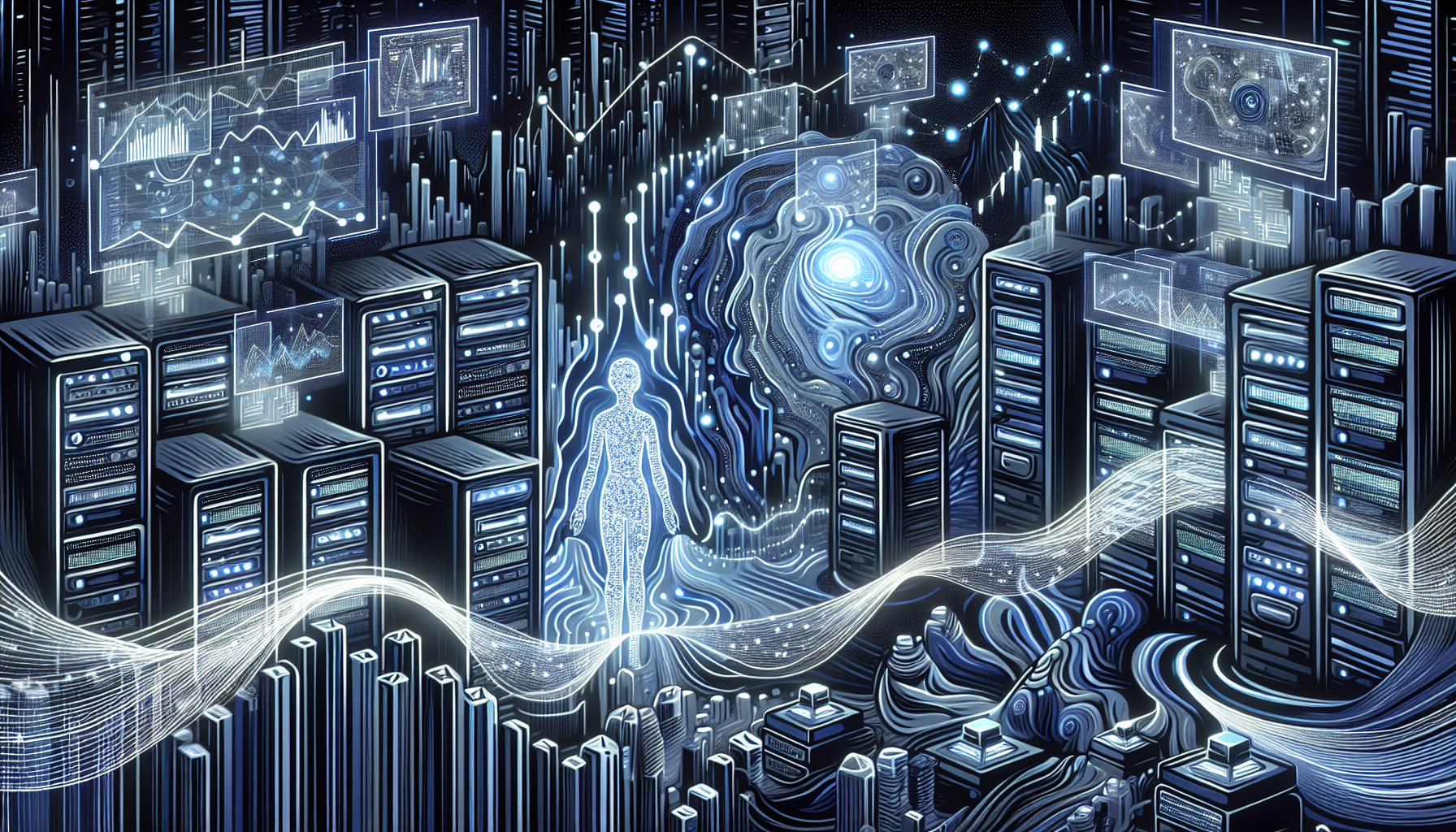
The algorithmic trading market is growing quickly. It’s expected to go from USD 11.1 billion in 2019 to USD 18.8 billion by 2024, with stocks playing a big role in this growth. Exchange-Traded Funds (ETFs), which are becoming a big part of the algorithmic trading market, are one area where this growth is especially clear.
Execution algorithms have changed a lot over the years. They used to be simple systems based on rules, but now they’re very complex and can look at real-time market data and find trading opportunities. The most recent innovations in this area are machine learning and artificial intelligence, which make it easier to analyze data and come up with complex trading strategies. Additionally, algorithmic trading makes it possible to study and test hypotheses in a variety of financial situations, which lowers risk without having to do actual trading on the market.
Machine Learning in Algo Trading
Machine learning is transforming algorithmic trading, enhancing its prowess in:
- Data analysis
- Pattern recognition
- Risk management
- Predictive modeling
This big step forward in technology makes it possible to combine signals from huge datasets, which helps people make decisions and gives them an edge in the market.
It can be hard to use machine learning in trading systems, though, because too much optimization can make models that work perfectly on paper but not in the unpredictable real-world markets. There is also a chance that using wrong or incomplete data sets could hurt the performance of trading algorithms, which could cause big losses.
Still, machine learning lets trading strategies change on the fly, which lets high-frequency trading algorithms change with the markets and make quick choices. More machine learning and AI will likely be used in execution algorithms in the future. This will allow for more complex data analysis and strategy development.
Companies like Two Sigma Investments show how machine learning can be used successfully in algorithmic trading by taking care of large amounts of money and making great returns every year.
The Evolution of Execution Algorithms
Execution algorithms have grown up like a good wine, making trade execution better, lowering slippage, and making trading better overall. They can adjust to changes in the market, which helps protect capital and get the best returns. In high-frequency trading, where choices need to be made in nanoseconds, these algorithms are able to keep up. Real-time assessments of the market allow for strategic order routing, which makes the most of small price differences to make money.
The first high-frequency trading strategies were made by proprietary trading firms in the late 1990s. This was the start of the evolution of execution algorithms. Here are some common execution algorithms:
- Percentage of Volume (POV): ensures orders are executed in a manner that aligns with market volume
- Volume-Weighted Average Price (VWAP): ensures orders are executed at price averages
- Time Weighted Average Price (TWAP): seeks to achieve a price close to the average over a specified timeframe
These strategies try to find the best balance between the pros and cons of immediate and delayed execution in order to keep execution costs as low as possible.
People are also noticing that cloud-based algorithmic trading solutions are easy to maintain, cost-effective, scalable, and good at managing trade data.
Real-World Examples of Algorithmic Trading
The fact that top hedge funds and institutional investors have been successful with algorithmic trading is proof of its power. For example, the D.E. Shaw Group has mastered the art of using its own proprietary algorithms to sift through huge datasets and find profitable investment opportunities. From now until 2021, it promises a net annual return of 13.9%. The Medallion Fund from Renaissance Technologies is known for its advanced mathematical and computer algorithms. From 1988 to 2020, it had an amazing average annual return of 39.1% after fees.
Companies like Citadel Advisors, Bridgewater Associates, and AQR Capital Management have also been very successful with complex algorithmic trading strategies. This shows that this technology has the potential to bring in a lot of money.
Getting Started with Algorithmic Trading
Those who are interested in algorithmic trading need to have a strong background in math, statistics, programming, and creativity. Because it is based on technology, knowing how to code or program is very helpful, especially for people with STEM degrees like electrical engineering, computer science, math, or science that involves numbers.
Many online courses and blogs offer structured learning and tips for using what you’ve learned in real life. This makes the complicated world of algorithmic trading easier for people who are just starting out to understand.
Educational Pathways
If you want to learn how to do algorithmic trading, you need to be disciplined about it. It is important to have a solid understanding of quantitative analysis or modeling, with a focus on learning about technical indicators and coming up with strategies for following trends. Books and articles like “Quantitative Trading” by Ernest P. Chan and “Inside the Black Box” by Rishi K. Narang are very helpful for learning about numbers and how markets work.
To be successful in this field, you have to keep learning, and you can use reliable educational resources to help you.
Choosing a Trading Platform
For algorithmic traders, picking the right trading platform is a very important step. Platforms should be able to handle trades quickly, give accurate real-time market data, and have trading fees that are competitive. Some platforms are designed to work only with certain types of markets. For example, MetaTrader, NinjaTrader, and cTrader are popular choices for trading forex and futures because they have advanced charting and automation tools.
A robust trading platform should offer the following features:
- User-friendly interface
- Real-time market data
- Advanced charting tools
- Order execution capabilities
- Backtesting functionalities to validate trading strategies against historical data
Platforms like TradeStation and QuantConnect are good for both new and experienced traders because they have a good mix of powerful tools and ease of use.
The Debate: Algorithmic Trading vs. Discretionary Trading
A lot of people have strong opinions about the pros and cons of algorithmic trading versus discretionary trading. Here are some important things to think about:
- Usually, algorithmic trading is more reliable and has lower execution costs than discretionary trading. This makes it more useful.
- The discipline of algorithmic trading makes trading decisions less based on emotion, which can lead to better risk management.
- Discretionary traders, on the other hand, may be better off when unexpected market events happen because their gut feelings and experience can work better than systems that are already set up.
- Discretionary traders can quickly change their strategies, which gives them an advantage over algorithmic systems that might need big changes or recoding to behave differently.
Setting up and maintaining algorithmic trading costs more, and because there are no emotional biases, it can miss the “gut feelings” that lead to big gains in discretionary trading. Both approaches are getting more and more attention from regulators who want to make sure the market is fair and clear. This has an effect on how trading strategies are made and used.
Statistical data of Algorithmic Trading Success
Statistical information can tell you a lot about how well algorithmic trading works. Here are some important facts:
- Algorithmic trading accounts for about 60-75% of overall trading volume in key financial markets and approximately 40% in emerging economies like India.
- The growing volume of algo-trading in the stock market is expected to contribute a significant $8.61 billion to the market share by 2027.
- In the Forex market, a staggering 92% of trading in 2019 was executed by algorithms rather than humans.
- Hedge funds in Europe and the U.S. have also increased their reliance on algo providers.
- The algorithmic trading market is expected to reach $18.8 billion by 2024, with North America leading the charge.
These figures underscore the growth trajectory of algorithmic trading.
A case study from a leading stockbroking firm in India highlights the efficacy of customized algorithmic trading software, achieving a success rate of up to 97% for their trading strategies.
Summary
With all the information we’ve learned about algorithmic trading, it’s become clear that it gives modern traders a lot of useful tools. Because of how precisely trades are executed and how strong risk management strategies are, algorithmic trading has become an important part of the financial markets. The success stories of institutional investors and the positive statistical data show that it has a lot of potential. But the way isn’t easy. It’s especially hard for small traders who have to deal with the high stakes and complicated technology of algorithmic systems. Despite these hurdles, the advancements in machine learning and AI herald a new era of trading algorithms that could redefine success in the markets.
Frequently Asked Questions
Can trading algorithms work?
Absolutely, trading algorithms can work well by making trading decisions quickly, efficiently, and objectively. Their ability to automate entry and exit points lowers the chance of mistakes and stops information from getting out.
How much money can you make with algorithmic trading?
While working as an algorithmic trader in the US, the average yearly pay is $85,750, or $41.23 per hour. This can be different depending on things like experience and how well the market is doing currently.
What is the success rate of algorithmic trading?
Although algorithmic trading has a mixed success rate, studies show that between 50 and 60 percent of strategies are profitable. This depends on things like the quality of the algorithm and the state of the market.
What is algorithmic trading?
Automated computer programs run trades based on set rules. This lets computers make quick, frequent trading decisions that are hard for humans to match.
How does algorithmic trading differ from traditional trading?
When you trade using algorithmic trading, computers make trades based on mathematical models and data analysis, which is faster, more accurate, and more consistent than humans making decisions.