How did history of algorithmic trading evolve from rule-based systems in the 1940s to state-of-the-art strategies powered by artificial intelligence (AI)? This historical account of algorithmic trading elucidates the pivotal shifts, technical developments, and regulatory interventions that have molded the application of algorithms in trading and, by extension, the contemporary financial environment. Along the way, learn about the turning points that have changed the game for market efficiency and speed while also presenting new obstacles for regulators and traders.
Key Takeaways
- Beginning with rule-based funds and Markowitz’s Modern Portfolio Theory, algorithmic trading has progressed to more complex strategies driven by artificial intelligence (AI) that span various asset classes.
- The expansion and incorporation of algorithmic trading in financial markets have been greatly impacted by electronic communication networks and landmark regulatory changes.
- The ever-evolving regulatory landscape is crucial for maintaining market stability and safeguarding investors, but developments in quantum computing, artificial intelligence, and blockchain technology have the potential to further improve algorithmic trading.
The Genesis of Algorithmic Trading
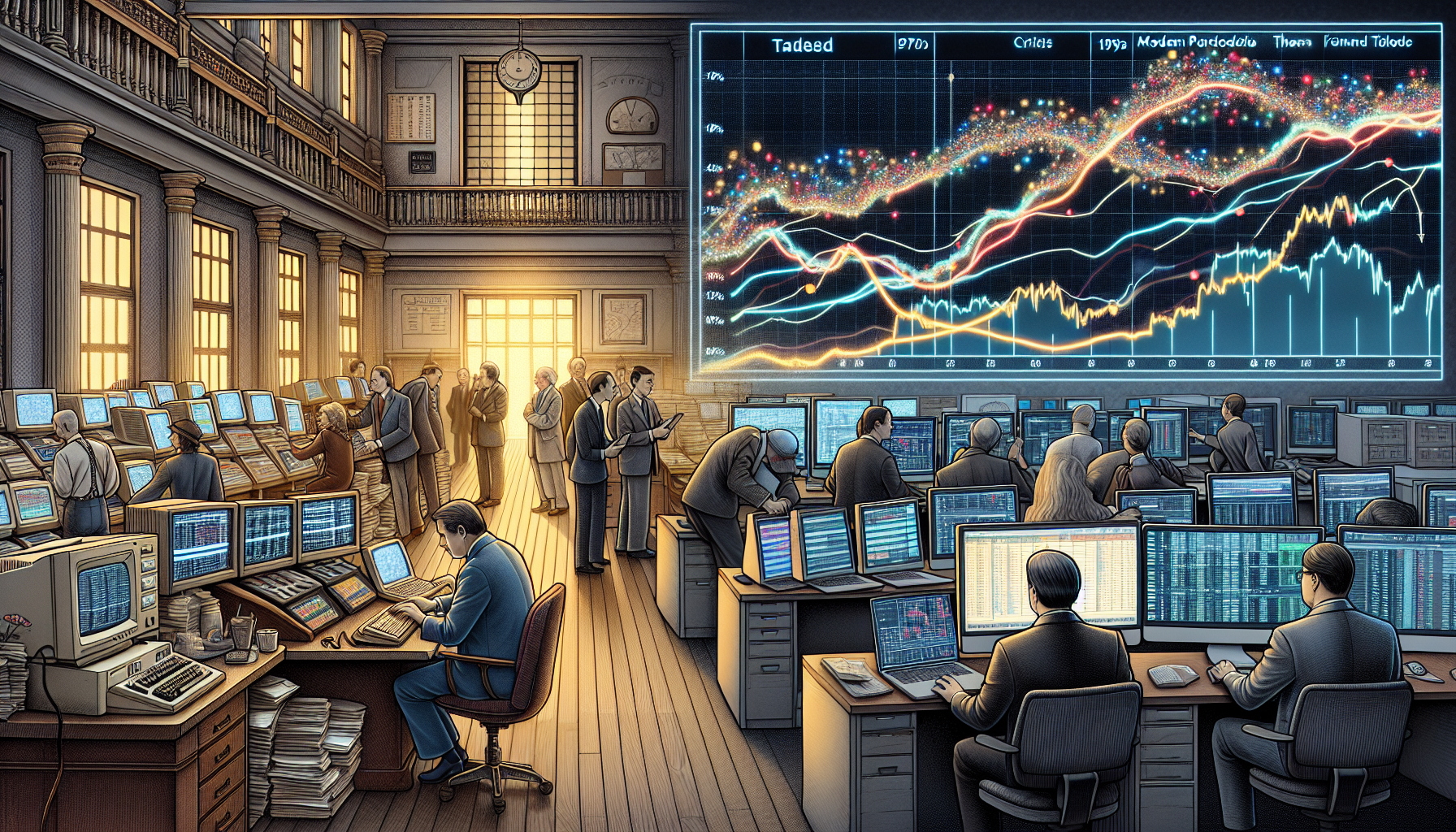
The concept of algorithmic trading had its origins in times before the advent of the digital era. One of the ancestors of modern algorithmic strategies, the first rule-based fund appeared in 1949. A year later, the guiding light of the emerging discipline of quantitative finance—Modern Portfolio Theory—would be introduced by Harry Markowitz. There was a dramatic shift in the way the financial markets functioned due to these preliminary advancements and the explosion of internet-based communication networks in the 1990s.
Financial institutions started to see automated trading more favorably as the century went on. In the 1970s, the first dynamic hedging strategies and simple algorithms to execute trades at favorable prices began to take shape. Algorithmic traders were already honing their techniques when the US decimalization process was finally finished in 2001, opening the door for small price differences between bid and offer prices. This was the era of development, the calm before the digital revolution that would shake up the trading world.
Rule-Based Funds and Modern Portfolio Theory
A fresh perspective on investment portfolios was required in the financial sector prior to the widespread adoption of algorithmic trading strategies. That fresh viewpoint was abundantly provided by Harry Markowitz’s seminal work on MPT, which established the essential principles of diversification and risk management. After laying out a mathematical framework that took into account an investor’s risk tolerance and reward expectations, Markowitz revolutionized the industry with the 1952 publication of “Portfolio Selection” by creating the ideal asset mix.
The evolution of investment strategies was greatly aided by the adoption of MPT. Investment risk assessment became more complex, and the emphasis moved from finding cheap stocks to building balanced portfolios, thanks to the Capital Asset Pricing Model. After years of focusing on specific stock selections rather than market trends, this was a huge change in investment strategy.
Emergence of Electronic Communication Networks
Due to the proliferation of ECNs, the 1990s were a watershed decade in the history of the financial markets. Thanks to these cutting-edge systems, which automatically match buy and sell orders, traders now have access to liquidity pools, multiple exchanges, and quicker execution times than before. Electronic trading was not only growing at the time; it was radically changing the trading environment.
Electronic trading systems were encouraged to be adopted across the financial infrastructure and a regulated framework was established with the implementation of Regulation ATS in 1998. There was a shift in the trading landscape with the advent of electronic communication networks (ECNs), paving the way for algorithmic traders to emerge and eventually rule the financial markets.
The Pioneers: Early Algorithmic Trading Systems
As the foundations of electronic trading were being laid, pioneering systems began to surface, setting the blueprint for the future of algorithmic trading. Some of these systems include:
- The NYSE’s Designated Order Turnaround (DOT) system, introduced in the early 1970s, which facilitated electronic order execution and bypassed the need for phone calls to brokers.
- The London Stock Exchange’s SEAQ (Stock Exchange Automated Quotation) system, introduced in 1986, which allowed for electronic trading and improved market transparency.
- The NASDAQ’s SuperMontage system, introduced in 2002, which consolidated and automated the trading process for NASDAQ-listed securities.
These systems were a sign of things to come, as the financial markets began to embrace the efficiencies of automated trading.
The 1980s saw a surge in the sophistication of trading algorithms. Automatic execution of lists of orders became possible, harnessing market conditions to the trader’s advantage. This era also witnessed the rise of program trading, especially in the form of index arbitrage strategies that played the S&P 500 equity and futures markets against each other. These early algorithmic trading systems were the trailblazers, setting the stage for the digital revolution that was just over the horizon.
NYSE’s Designated Order Turnaround System
The New York Stock Exchange’s DOT system was a significant leap forward, introducing a level of speed and convenience previously unimaginable. By electronically transmitting orders, traders could bypass the traditional, time-consuming phone calls to brokers, allowing for a more streamlined and efficient process. This system would later evolve into the SuperDOT, capable of handling even larger orders, marking a new era in the york stock exchange and reinforcing the NYSE’s position as a major financial hub for stock trades.
The significance of the DOT system lay not only in its immediate impact but also in the precedent it set for future electronic trading systems. It was a clear indication that the financial industry was ready to:
- automate trading
- embrace the potential of algorithmic trading
- offer online trading solutions that could handle the increasing trading volume of the stock market.
Instinet Trading Platform
In 1969, a significant milestone was reached with the launch of the Instinet trading system. This platform allowed institutional investors, such as hedge funds and investment banks, to trade stocks outside the traditional exchanges, heralding a new age in stock market accessibility.
Instinet was revolutionary, providing:
- a trading platform
- real-time market data
- a market data system that empowered traders with the information they needed to make informed trading decisions.
Instinet’s significance lies not only in its own trading algorithms but also in the way it paved the path for future online trading platforms. It demonstrated the viability and demand for alternative trading systems, which could offer investors direct access to the financial markets without the need for a traditional stock exchange as the proper trading post.
The Digital Revolution: Advancements in Technology and Strategy
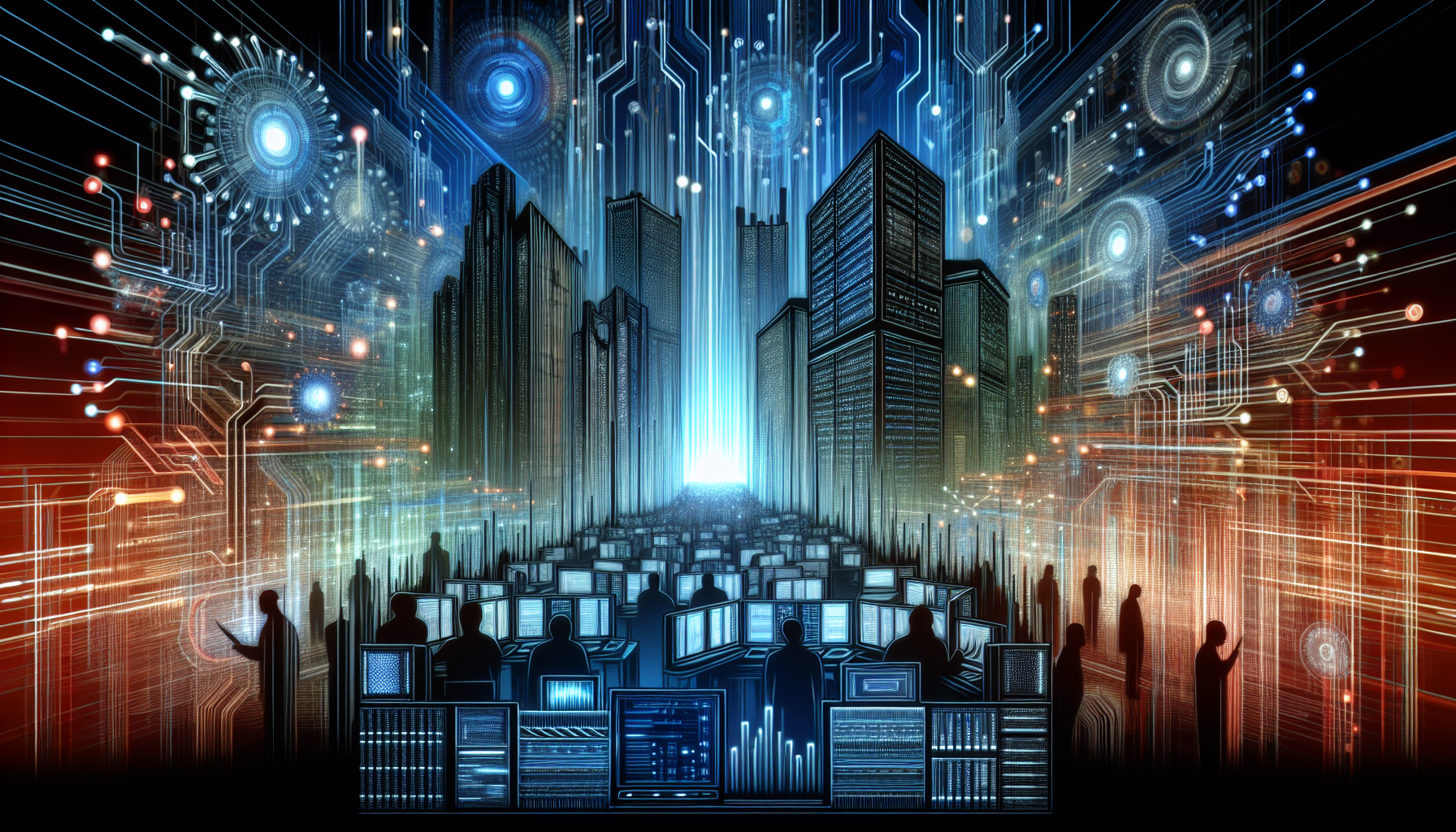
As the 20th century waned, a digital revolution took hold of the financial industry. The replacement of open outcry systems with electronic trading systems in the late 1990s marked a turning point, opening up global markets to algorithmic traders. The subsequent growth in data availability and processing power in the 2000s fueled the development of advanced algorithms and trading strategies that could exploit these new opportunities.
This period saw:
- The rise of direct market access
- The explosive growth of high-frequency trading (HFT), which leveraged ultra-fast execution speeds to capitalize on market opportunities within microseconds
- The integration of machine learning and artificial intelligence into trading algorithms, leading to real-time adaptation to market conditions
Rise of Direct Market Access and High-Frequency Trading
The internet boom of the 1990s was a catalyst for change, facilitating the evolution of high-frequency trading (HFT) that would come to dominate the market in the following decades. The authorization of electronic exchanges by the SEC in 1998 created a landscape ripe for HFT practices, allowing firms to offer online trading and execute trades electronically with unprecedented speed and efficiency. By 2012, HFT accounted for around 70% of all equity trading volume in the US, highlighting its dominance in the financial industry.
HFT strategies are not just about speed; they also rely on:
- Analyzing real-time market data
- Executing trades based on this analysis
- Integrating big data and machine learning to become more dynamic and adapt to market trends in real-time
- Using natural language processing (NLP) algorithms to parse news and make trading decisions based on sentiment analysis.
Integration of Machine Learning and Artificial Intelligence
Artificial intelligence and machine learning have been game-changers for algorithmic trading, providing a level of sophistication and adaptability that was previously unattainable. These technologies have enabled the identification of complex patterns and improved the prediction of market movements, allowing trading strategies to adapt and evolve with the market. The future is even more promising, with advancements in deep learning expected to refine the accuracy of these predictions further.
The potency of AI in algorithmic trading lies in its ability to leverage unstructured data, such as social media and news articles, and turn it into actionable trading signals. Predictive analytics and sentiment analysis are becoming increasingly important tools for algorithmic traders, enabling them to forecast market trends and make more informed trading decisions.
Challenges and Controversies: Algorithmic Trading’s Impact on Financial Markets
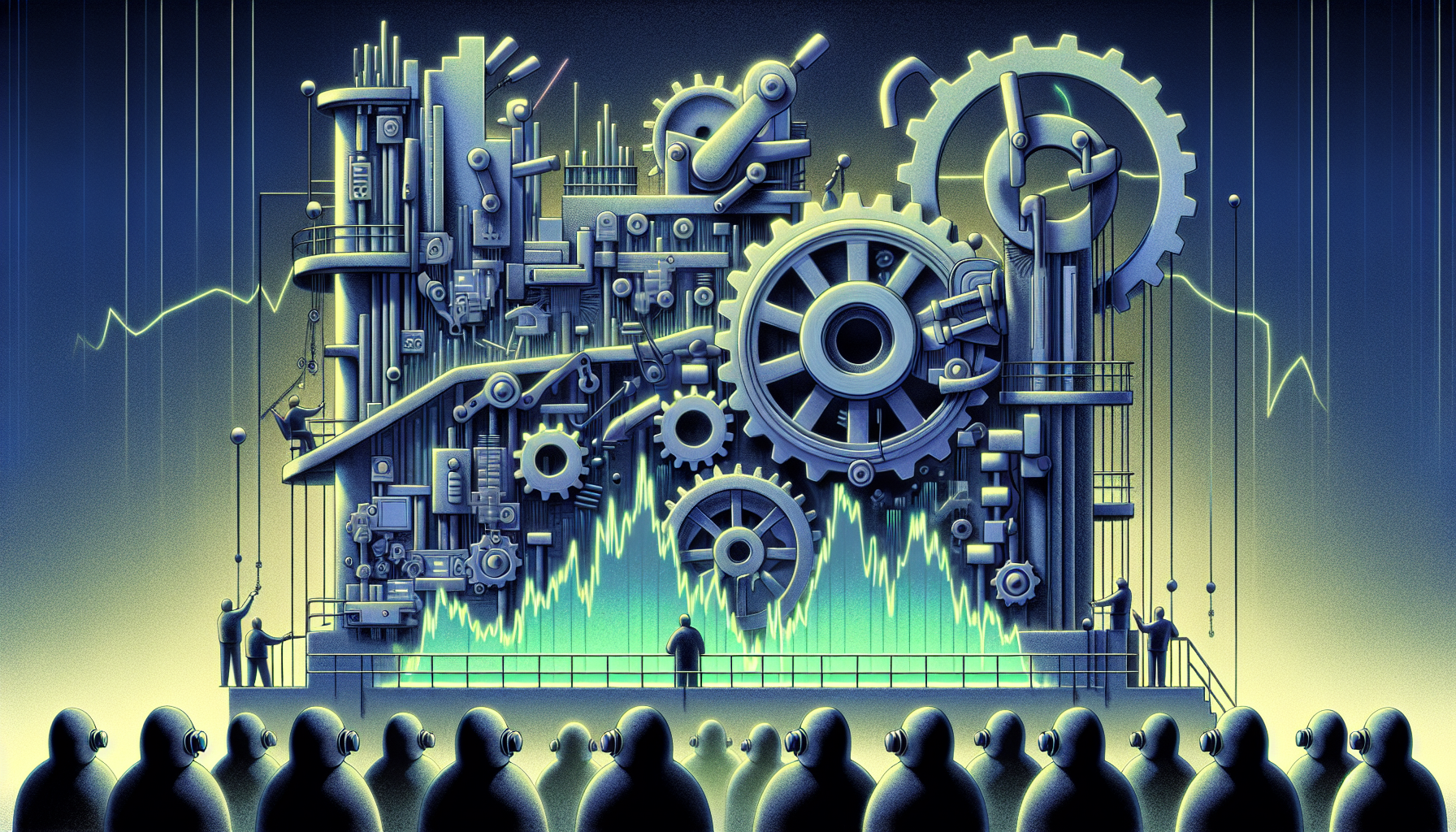
Despite the successes and advancements of algorithmic trading, it has not been without its challenges and controversies. Algorithmic strategies can lead to:
- Herding behavior and feedback loops, reinforcing market trends and contributing to volatility.
- Ethical concerns, such as potential job displacement and the capacity for ethical decision-making in automated trading systems.
- Market manipulation, with new techniques being used to influence market prices or create artificial conditions.
The integration of AI in trading has raised these concerns and more.
Regulators have found themselves in a constant race to keep up with the rapid speed and complexity inherent in algorithmic trading. This struggle has led to new challenges in maintaining market stability and the prevention of market crashes. The role of algorithmic trading in market events has drawn scrutiny and raised questions about the need for enhanced oversight and regulation.
The 2010 Flash Crash
One of the most significant events that brought algorithmic trading into the public spotlight was the 2010 Flash Crash. This event highlighted the potential for algorithmic trading, particularly HFT, to impact market stability. High-frequency traders, using advanced programs capable of executing trades at incredibly high speeds, were identified as key contributors to the crash, alongside complex derivatives. The use of strategies such as quote stuffing by high-frequency traders was also scrutinized, as it was seen to create artificial latencies and arbitrage opportunities.
The aftermath of the Flash Crash led to an investigation by the U.S. Securities and Exchange Commission and the Commodity Futures Trading Commission, which confirmed the role of both algorithmic trading and HFT in the volatility experienced during the event. This event was a wake-up call, highlighting the need for a better understanding and regulation of these advanced trading strategies.
Regulatory Responses
In the wake of the Flash Crash and other market turbulences, regulators stepped up to introduce measures to ensure fairness and transparency in algorithmic trading. The Dodd-Frank Wall Street Reform and Consumer Protection Act, for instance, was enacted to enhance market transparency, regulate derivatives, and strengthen financial institutions’ oversight, directly affecting the operation of algorithmic trading. Across the pond, MiFID II in the European Union introduced stricter rules on investor protection, market transparency, and structure, affecting the operation of financial markets, including algorithmic trading.
These regulatory responses underscored the need for robust risk management systems in the context of algorithmic trading, aiming to prevent activities that could undermine the integrity of financial markets. Regulators recognized that with the continuous evolution of financial markets and trading strategies, a dynamic regulatory framework was necessary to ensure market stability and protect investors.
Present Landscape: Algorithmic Trading in Today’s Market
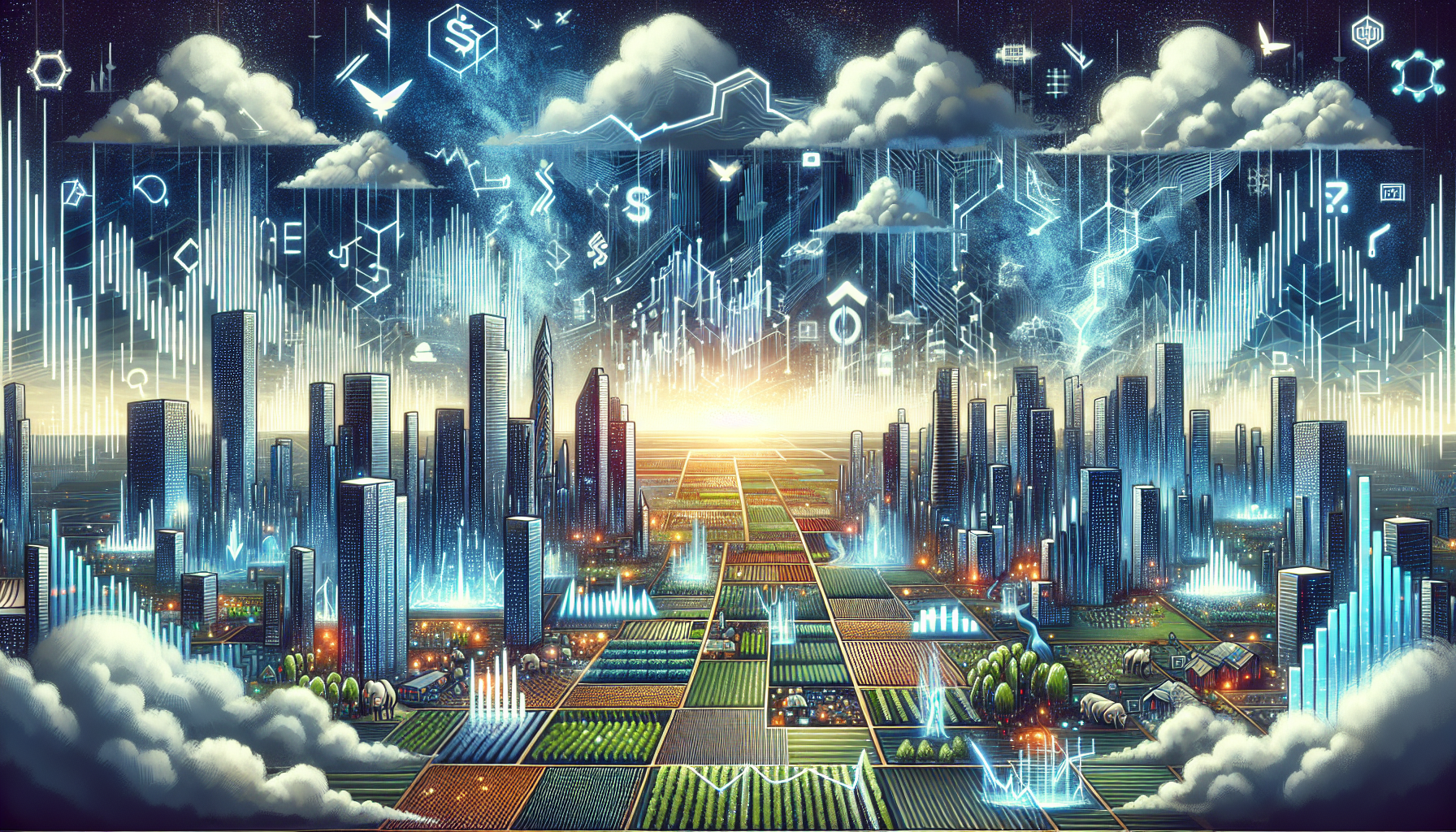
Today, algorithmic trading is not just confined to the equity markets but has expanded across a wide array of asset classes, including derivatives, commodities, and the forex market. The presence of trading platforms with powerful APIs has catalyzed the growth of algorithmic trading, especially in markets like India, offering traders sophisticated automated trading strategies. Additionally, the integration of AI and machine learning has significantly improved the efficiency of these trading systems.
Algorithmic trading has had a significant impact on market liquidity, providing one of its notable benefits. This has helped in enhancing the ease and efficiency of trading in the market. By providing a continuous stream of buy and sell orders, algorithmic trading helps maintain market prices and facilitate smoother transactions. This, in turn, contributes to the overall efficiency of the markets, allowing for the seamless execution of trades and the management of market value.
Algorithmic Trading Across Asset Classes
Algorithmic trading’s influence extends beyond traditional stock exchanges into asset classes like the forex market, where, as of 2019, around 92% of all trading was performed by algorithms. The strategies employed in each asset class take into account their unique attributes and dynamics. For example, derivatives offer complex instruments that provide unique opportunities for algorithmic trading to exploit.
The development of these strategies is not just about applying a one-size-fits-all approach. Each market has its own set of technical indicators and market movements that must be considered. By tailoring these strategies to the specific needs of each asset class, algorithmic traders can maximize their effectiveness and adapt to the shifting tides of the global financial markets.
Rising Popularity of Algorithmic Trading Platforms
The allure of algorithmic trading has been greatly amplified by the rising popularity of dedicated trading platforms. These platforms, like Gridcap.us, equip traders with a comprehensive suite of tools, including live market data feeds, individualized watchlists, and customizable chart types, providing a robust framework for executing advanced trading strategies. The platforms not only offer automated verification and onboarding but also present limitless customization capabilities for personalized trading workspaces, catering to the diverse needs of algorithmic traders.
With the integration of real-time news content from sources like social media, platforms such as Dataminr have become instrumental in generating actionable trading signals using AI and machine learning. Moreover, the extension of services to support cryptocurrency trading by platforms like Gridcap.us is a testament to the evolving nature of the markets and the continuous innovation within the algorithmic trading landscape.
The Future of Algorithmic Trading: Trends and Predictions
Peering into the future of algorithmic trading reveals a landscape ripe with potential, driven by relentless advancements in technology. The future of algorithmic trading is expected to be shaped by the following:
- Quantum computing: The emergence of quantum computing is expected to provide a quantum leap in computational capabilities, opening the door to even more complex and accurate trading strategies.
- Blockchain technology: Blockchain technology is set to play a pivotal role in algorithmic trading, promising enhanced security, transparency, and reliability in trade execution.
- Advancements in AI: Further advancements in AI will contribute to the development of more sophisticated and personalized algorithmic trading strategies, tailored to individual risk profiles and preferences.
This integration of emerging technologies points to a future where algo trading and algorithmic trading strategies are more sophisticated, personalized, and tuned to individual risk profiles and preferences.
The industry’s trajectory is unmistakably forward, with high expectations for algorithmic trading to maintain its significant role in the financial markets. High-frequency trading and news-based trading, in particular, are areas where ground-breaking developments are anticipated. With the potential for greater integration of news analysis with algorithmic trading and quantitative techniques, the future looks bright for these advanced trading strategies.
Machine Learning and Artificial Intelligence Advancements
The engines driving the future of algorithmic trading are undoubtedly machine learning and AI. As these technologies evolve, machine learning algorithms in trading are expected to become increasingly adaptive, optimizing strategies in real-time to reflect changing market conditions. The self-improving capabilities of these algorithms signify a future where trading strategies are not just static sets of rules but dynamic systems that learn and evolve.
Predictive analytics powered by AI is set to enable traders to anticipate market movements with greater precision, offering a significant edge in the competitive world of trading. AI is anticipated to progress beyond just analyzing and executing trades based on market data, moving towards providing in-depth insights across different financial domains. These advancements will empower traders to make more nuanced and informed trading decisions, leveraging the full potential of algorithmic trading.
Regulatory Landscape
As algorithmic trading evolves, so too must the regulatory landscape. Effective regulatory oversight and communication about the benefits and risks associated with algorithmic trading are crucial for shaping public trust and guiding the industry’s development.
As we look ahead, regulators will need to:
- Strike a balance between fostering innovation and ensuring market stability
- Update regulations to keep pace with the industry’s rapid advancement
- Implement ongoing monitoring to detect and address any potential risks
- Explore the use of new technological tools to enhance regulatory oversight
By taking these steps, regulators can ensure that algorithmic trading continues to thrive while also safeguarding the integrity and stability of the financial markets.
The future of regulation in algorithmic trading may also see a greater emphasis on:
- Collaboration between regulators and industry participants
- Developing standards and practices that safeguard the integrity of financial markets
- Encouraging the responsible use of algorithmic trading strategies
This cooperative approach could be key to managing the complexities of modern financial systems and maintaining a fair and efficient market for all participants.
Summary
As we have journeyed through the annals of algorithmic trading, from its inception to the cutting-edge of today’s markets, it’s clear that this financial practice has not only evolved but revolutionized the way trading is conducted. Algorithmic trading has moved from the domain of a select few to a widespread tool that enhances market efficiency and liquidity. Along the way, it has faced its share of challenges and controversies, prompting a wave of regulatory measures to ensure market stability and fairness.
The future of algorithmic trading is bright, teeming with possibilities brought about by advancements in AI, machine learning, and emerging technologies like blockchain and quantum computing. As this future unfolds, the industry must continue to adapt, innovate, and collaborate with regulators to ensure that the benefits of algorithmic trading can be realized by all market participants. The path forward is one of mindful progression, where the pursuit of technological excellence is balanced with the imperative to uphold market integrity and foster investor confidence.
Frequently Asked Questions
What percentage of trading is algorithmic?
About 60-75% of overall trading volume in the U.S. equity market, European financial markets, and major Asian capital markets is accounted for by algorithmic trading. This method uses pre-programmed strategies executed without direct human intervention.
What is the history of automated trading system?
Automated trading systems have a history dating back to the 1970s, when basic algorithms were used to execute trades on stock exchanges. However, the concept was first introduced by Richard Donchian in 1949 as a way to autonomously initiate trades based on predetermined market conditions.
Who is the founder of algorithmic trading?
The founder of algorithmic trading is Thomas Peterffy, who started the practice of sending coded instructions from a broker’s computer to an exchange’s terminal using a typing cyborg.
How have electronic communication networks affected algorithmic trading?
The use of Electronic Communication Networks (ECNs) has significantly impacted algorithmic trading by improving trade execution speed, providing access to more trading opportunities, and connecting traders to multiple exchanges and liquidity pools. This infrastructure was crucial for the expansion of algorithmic trading.
What was the impact of the 2010 Flash Crash on algorithmic trading?
The 2010 Flash Crash raised concerns about the stability of financial markets due to advanced algorithmic trading strategies, leading to increased scrutiny and regulatory measures. This event highlighted the need for safeguards to prevent similar occurrences.